Lars van der Laan
University of Washington, Seattle. Department of Statistics
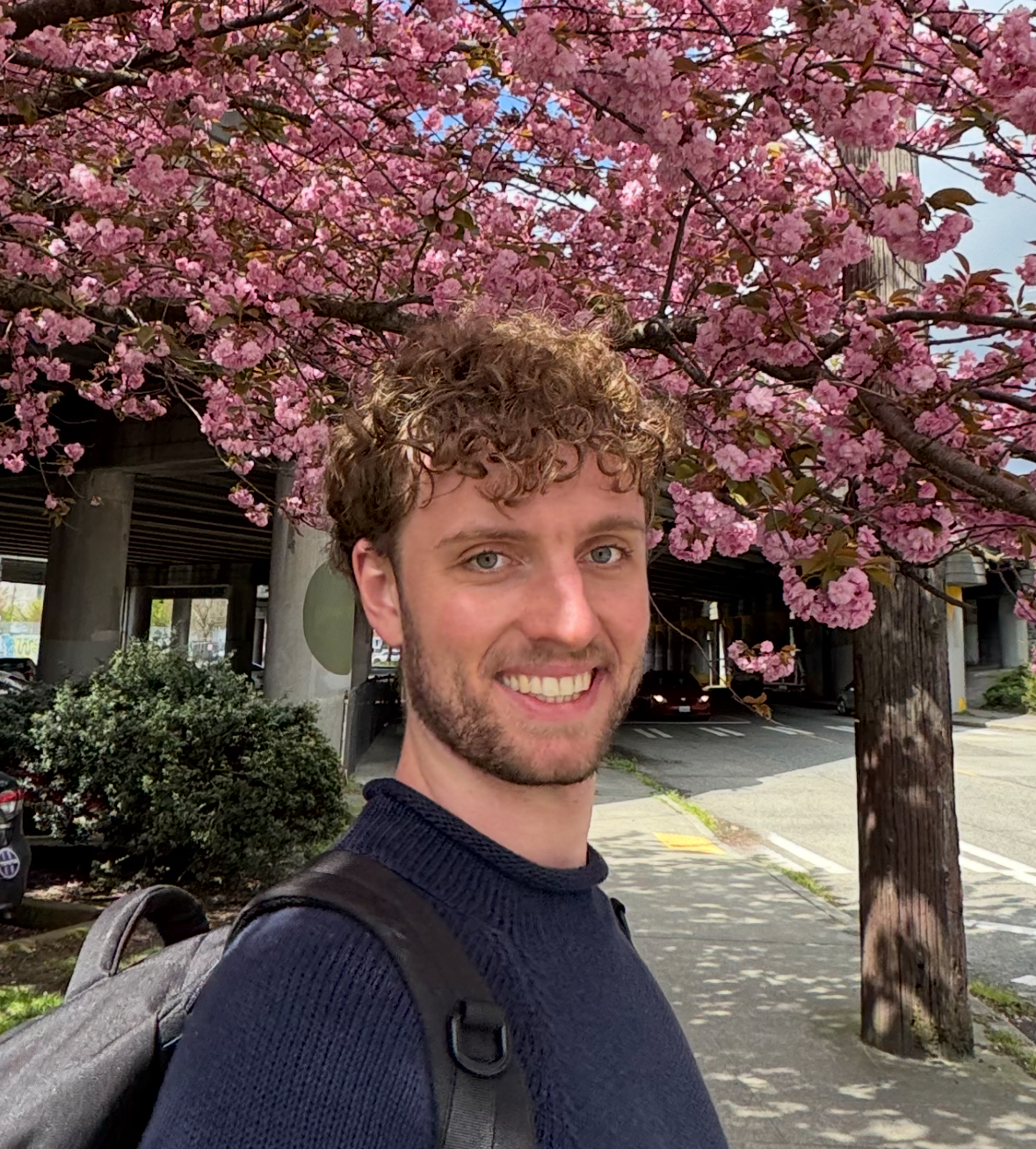
B313
Padelford Hall, Northeast Stevens Way
Seattle, WA 98103
lvdlaan@uw.edu
About me
I am a fourth-year Ph.D. student in Statistics at the University of Washington, advised by Marco Carone and Alex Luedtke.
My research focuses on causal inference, statistical learning, and semiparametric efficiency theory.
I develop methods for debiased and efficient estimation using modern machine learning tools, including doubly robust inference, simplifying inference via automatic debiasing, inference after model selection, and estimation of heterogeneous treatment effects.
A central theme of my research is bridging calibration—a tool traditionally used in prediction—with causal inference. This includes methods such as causal isotonic calibration for heterogeneous treatment effects, stabilized inverse probability weighting, Bellman calibration for reinforcement learning, and calibrated debiased machine learning.
I also work on distribution-free uncertainty quantification in predictive settings. As a Visiting Student Researcher at UC Berkeley, I collaborate with Ahmed Alaa, with peer-reviewed work presented at NeurIPS and ICML. This includes integrating calibration into conformal prediction and developing generalized Venn–Abers calibration for uncertainty-aware prediction.
I am currently supported by a Netflix Graduate Research Fellowship, and work with Nathan Kallus and Aurélien Bibaut on sequential and long-term causal inference and nonparametric instrumental variables inference.
I’ve applied my research in causal inference and machine learning to problems in both biomedical and technology domains, including internships at Genentech, the Fred Hutchinson Cancer Center, and Netflix. I am also a contributor to the tlverse software ecosystem and consult on software development for TLRevolution.
I have also served as a teaching assistant for graduate-level courses in theoretical statistics and I enjoy mentoring students through research projects.